This course provides an introduction to supervised models, unsupervised models, and association models. This is an application-oriented course and examples include predicting whether customers cancel their subscription, predicting property values, segment customers based on usage, and market basket analysis.
Argomenti
Introduction to machine learning models- Taxonomy of machine learning models- Identify measurement levels- Taxonomy of supervised models- Build and apply models in IBM SPSS ModelerSupervised models: Decision trees - CHAID- CHAID basics for categorical targets- Include categorical and continuous predictors- CHAID basics for continuous targets- Treatment of missing valuesSupervised models: Decision trees - C&R Tree- C&R Tree basics for categorical targets- Include categorical and continuous predictors- C&R Tree basics for continuous targets- Treatment of missing valuesEvaluation measures for supervised models- Evaluation measures for categorical targets- Evaluation measures for continuous targetsSupervised models: Statistical models for continuous targets - Linear regression- Linear regression basics- Include categorical predictors- Treatment of missing valuesSupervised models: Statistical models for categorical targets - Logistic regression- Logistic regression basics- Include categorical predictors- Treatment of missing valuesSupervised models: Black box models - Neural networks- Neural network basics- Include categorical and continuous predictors- Treatment of missing valuesSupervised models: Black box models - Ensemble models- Ensemble models basics- Improve accuracy and generalizability by boosting and bagging- Ensemble the best modelsUnsupervised models: K-Means and Kohonen- K-Means basics- Include categorical inputs in K-Means- Treatment of missing values in K-Means- Kohonen networks basics- Treatment of missing values in KohonenUnsupervised models: TwoStep and Anomaly detection- TwoStep basics- TwoStep assumptions- Find the best segmentation model automatically- Anomaly detection basics- Treatment of missing valuesAssociation models: Apriori- Apriori basics- Evaluation measures- Treatment of missing valuesAssociation models: Sequence detection- Sequence detection basics- Treatment of missing valuesPreparing data for modeling- Examine the quality of the data- Select important predictors- Balance the data
Obiettivi
Introduction to machine learning models - Taxonomy of machine learning models - Identify measurement levels - Taxonomy of supervised models - Build and apply models in IBM SPSS Modeler
Supervised models: Decision trees - CHAID - CHAID basics for categorical targets - Include categorical and continuous predictors - CHAID basics for continuous targets - Treatment of missing values
Supervised models: Decision trees - C&R Tree
- C&R Tree basics for categorical targets - Include categorical and continuous predictors - C&R Tree basics for continuous targets - Treatment of missing values
Evaluation measures for supervised models - Evaluation measures for categorical targets - Evaluation measures for continuous targets
Supervised models: Statistical models for continuous targets - Linear regression - Linear regression basics - Include categorical predictors - Treatment of missing values
Supervised models: Statistical models for categorical targets - Logistic regression - Logistic regression basics - Include categorical predictors - Treatment of missing values
Association models: Sequence detection - Sequence detection basics - Treatment of missing values
Supervised models: Black box models - Neural networks - Neural network basics - Include categorical and continuous predictors - Treatment of missing values
Supervised models: Black box models - Ensemble models - Ensemble models basics - Improve accuracy and generalizability by boosting and bagging - Ensemble the best models
Unsupervised models: K-Means and Kohonen - K-Means basics - Include categorical inputs in K-Means - Treatment of missing values in K-Means - Kohonen networks basics - Treatment of missing values in Kohonen
Unsupervised models: TwoStep and Anomaly detection - TwoStep basics - TwoStep assumptions - Find the best segmentation model automatically - Anomaly detection basics - Treatment of missing values
Association models: Apriori - Apriori basics - Evaluation measures - Treatment of missing values
Preparing data for modeling - Examine the quality of the data - Select important predictors - Balance the data
Prezzo di listino
1.400,00 EUR + IVA per partecipante
Durata
- 16 ore
- 2 giorni
Prerequisiti
- Knowledge of your business requirements
Durata: 16 ore (2 giorni)
Erogabile on-line e on-site
Tutti i nostri corsi sono erogabili anche in modalità on-line (con formazione a distanza), oppure on-site, sempre personalizzati secondo le esigenze.
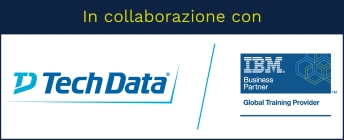